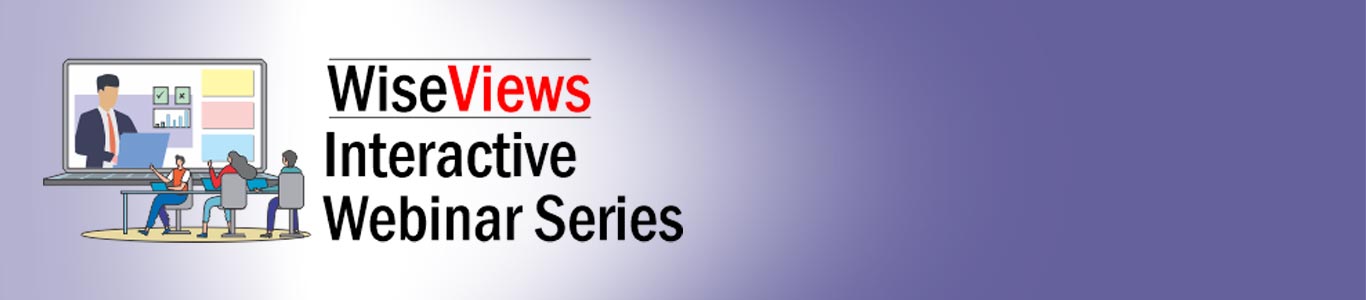
On Friday, January 13, 2023, we had a fascinating session by Prof Praveen Kopalle of the Tuck School of Business. Prof Kopalle spoke about the use of AI in marketing with special reference to customer reviews.
About Mr. Praveen Kopalle
Prof Praveen Kopalle is the Signal Companies’ Professor of Management and Professor of Marketing and the Chair of the marketing area at the Tuck School of Business, Dartmouth College. He received his Ph.D. from Columbia University, MBA from IIM Bangalore and B.E. from University College of Engineering, Osmania University. His teaching and research interests include innovation, pricing, e-commerce, machine learning, and artificial intelligence.
Professor Kopalle serves as an Associate Editor at the Journal of Marketing and Journal of Retailing and was an Associate Editor at the Journal of Consumer Research and International Journal of Research in Marketing. His current and past editorial board memberships include Journal of Consumer Research, Journal of Marketing Research, Journal of Marketing, Marketing Science, International Journal of Research in Marketing, Journal of Retailing, Production and Operations Management, Marketing Letters, Journal of Interactive Marketing, Journal of Revenue and Pricing Management, and International Journal of Electronic Commerce.
Professor Kopalle has won many awards including: IIM-Bangalore’s Distinguished Alumni Award, American Marketing Association’s Lifetime Achievement from the Retailing and Pricing SIG, Core Teaching Excellence Award at the Tuck School, John D. C. Little Best Paper Award in Marketing Science, Best Paper Award on Marketing and Innovation at the International Journal of Research in Marketing and William R. Davidson Award at the Journal of Retailing.
Prof Kopalle’s research has appeared in top-tier journals including Journal of Consumer Research, Journal of Marketing Research, Journal of Marketing, Marketing Science, Management Science, International Journal of Research in Marketing, Strategic Management Journal, JAMS, OBHDP, Journal of Retailing, Production and Operations Management, JPIM, Managerial and Decision Economics, Marketing Letters, Applied Economics, and International Journal of Electronic Commerce.
Prof Kopalle has spoken at over sixty universities and institutes worldwide, and is on the boards of IIMB Review, Management and Business Review, AtenWorks Inc., and Camelia Clothing Ltd, India.
About his research on customer reviews
Prof Kopalle spoke at length about his (done along with colleagues) research on customer reviews. Online product reviews are helpful to consumers for learning about product quality and fit and then making purchase decisions.
Firms are also concerned about the effect of reviews on sales and actively engage in review management. Much of the literature has focused on reviews and opinions written by non-expert consumers, experts, and managers of businesses. These reviews are written by humans.
Prof Kopalle and his colleagues have turned their attention to the task of the writing of product reviews by machines, i.e., through the use of tools and techniques of artificial intelligence algorithms. They trained a model through the linkage of product review text to a set of features of the product (the “metadata”). As a result of this training, the model can write new reviews given new (and thus, unseen) metadata.
The key research questions Prof Kopalle and his colleagues have tried to answer are:
- Can a machine generated review serve as a first draft for the expert reviewer?
- Can a machine generated summary of reviews ease the customer’s search costs?
Methodology
Prof Kopalle and fellow researchers trained their program on a decade’s worth of professional reviews—about 125,000 total—scraped from the magazine Wine Enthusiast. They also used nearly 143,000 beer reviews from the Web site RateBeer. The algorithm processed these human-written analyses to learn the general structure and style of a review. The algorithm was given a specific wine’s or beer’s details, such as winery or brewery name, style, alcohol percentage and price point. Based on these parameters, the algorithm found existing reviews for that beverage, pulled out the most frequently used adjectives and used them to write its own description.
The researchers selected one human and one AI-generated review each for 300 different wines and 10 human reviews and one AI review each for 69 beers. Then they asked a group of people to read both machine-generated and human-written reviews and checked whether the subjects could distinguish which was which. In most cases, they could not.
To generate syntheses of human-generated reviews, Prof Kopalle and his colleagues made use of a Transformer-based deep learning neural net architecture. This architecture is built on two main components: an encoder and a decoder. As per the standard practice in machine learning, the data was split into training and test sets. The former was used to tune the Transformer-based model. Test set data was not seen by the model before and was used to validate the model.
Key findings of the research
- Turing Tests show that machines can indeed learn to write “human-quality” reviews. Humans are unable to distinguish between human-written and machine-written reviews.
- The review produced by the machines could either be used as is or as a near-to-complete draft for a retailer to share with consumers.
- AI can summarize reviews of products quite effectively. Humans agree that the summary review captures ideas present in all the reviews.
- It is possible to create a gestalt review for products with thousands of reviews on Amazon.
- For products with little or no reviews, transformer technology may embed learning from reviews of similar products and provide a machine generated review.
Q&A
On his career lessons
There is no substitute for hard work. Short cuts do not work. Success involves 99% perspiration and 1% inspiration.
We should be committed to Plan A and not have a Plan B. We should give our 100% to Plan A. If we also have a Plan B from the start, we are setting ourselves up for failure.
The destination should not be our focus. The journey is more important. We should concentrate less on the outcome and more on the process.
On the use of AI in improving customer satisfaction
AI can be used to make products better. Customers are often not able to articulate their pain points clearly. They are used to doing things in a particular way. This where AI comes in. AI can observe people in their natural habitats and provide valuable inputs that can improve the design of products and services. This falls in the domain of Design Thinking. Another area is ‘conjoint design” which explores the trade-offs between different attributes. AI can be used to great advantage.
On going beyond artificial intelligence
Which one is superior, AI or Human Intelligence? Is the notion of singularity paramount? AI plus Human intelligence is superior. It is not OR but it is AND. Take the case of airlines, which often overbook flights to compensate for no shows by passengers, etc. When more number of passengers, than who can be accommodated, show up, they give incentives so that some passengers agree to board the next flight. In one celebrated case, there was one extra passenger. But no customer wanted to opt for the next flight and the crew forcibly made one passenger leave the plane. This incident attracted a lot of negative publicity. When the CEO was interviewed, he maintained that the crew was only doing their job. This is an example of a situation where AI cannot help, and human intelligence must come into play. In general, when there is a service failure and the customer is unhappy, leaders must display three behaviours:
- Apologise unconditionally without admitting guilt.
- Show empathy and tell the customer that they feel his/her pain.
- State sincerely that something will be done to address the problem.
On relating reviews to attributes
The essence of AI is making predictions. The prediction will be as good as the learning. Thus in case of autonomous trucks on highways, the learning will be more effective, compared to driverless cars on congested roads. So training the algorithm is important.
In case of wine or beer, the product can be decomposed into attributes. For example, in Prof Kopalle’s research, wine has 16 attributes. With training, reviews can be built around these attributes.
On ChatGPT
The biggest impact will be on educators. Suppose we ask students to write an essay on Shakespeare. How do we find out if the essay has been written by the student or by ChatGPT?
Earlier, while evaluating the homework of students, we would look at the handwriting. With the emergence of Word software, this became an irrelevant parameter. With the emergence of ChatGPT, we will have to come up with more innovative grading criteria. These criteria must be based on why we are making the students write the essay.
The real purpose of education is not so much to teach concepts as to provide the intuition behind the concept and explain the possible applications. So the biggest contribution of educators is to provide original, thoughtful and useful content. In the age of ChatGPT, educators will have to reinvent themselves.
On foreign universities entering India
Can we use AI to decide whether this is a good move? Since we do not have a past track record, we only have opinions. So, the algorithm can only summarise the opinions of experts.
Porf Kopalle feels that the entry of foreign universities will create more options for Indian students. For example, a student can choose between going to Stanford University in the US or attend Stanford’s classes in its campus in India. On balance, it is a good idea to allow foreign universities to enter India.
On the use of AI in India
India is rapidly emerging as a technology powerhouse. But the concern is that India is currently taking algorithms developed in the US and using them in India. The real opportunity lies in customizing them to the Indian context. India is a far more heterogeneous country compared to the US and it makes sense to tweak the algorithms to suit our needs.
On prediction and judgment
In many uncertain situations, there are two different dimensions at play: Prediction and Judgment. AI is better at making predictions. Human beings are better at making judgments. Prof Kopalle gave two examples to illustrate the point.
Earlier, we would select some goods and Amazon would ship them to us. Increasingly, Amazon will predict what we need and ship goods to us without waiting for orders. We can then decide what we want and reject the remaining items.
Spotify provides a selection of songs to Prof Kopalle. He decides which songs to accept or which to reject. Working this way, the cognitive load on the brain is reduced, time is saved and yet human judgment can be exercised so that we get exactly what we want.
Combining AI and human intelligence will give us the best results than either one alone. That is what matters for Prof Kopalle. Concerns about singularity taking over the world or machines getting the better of human beings are less important for him.